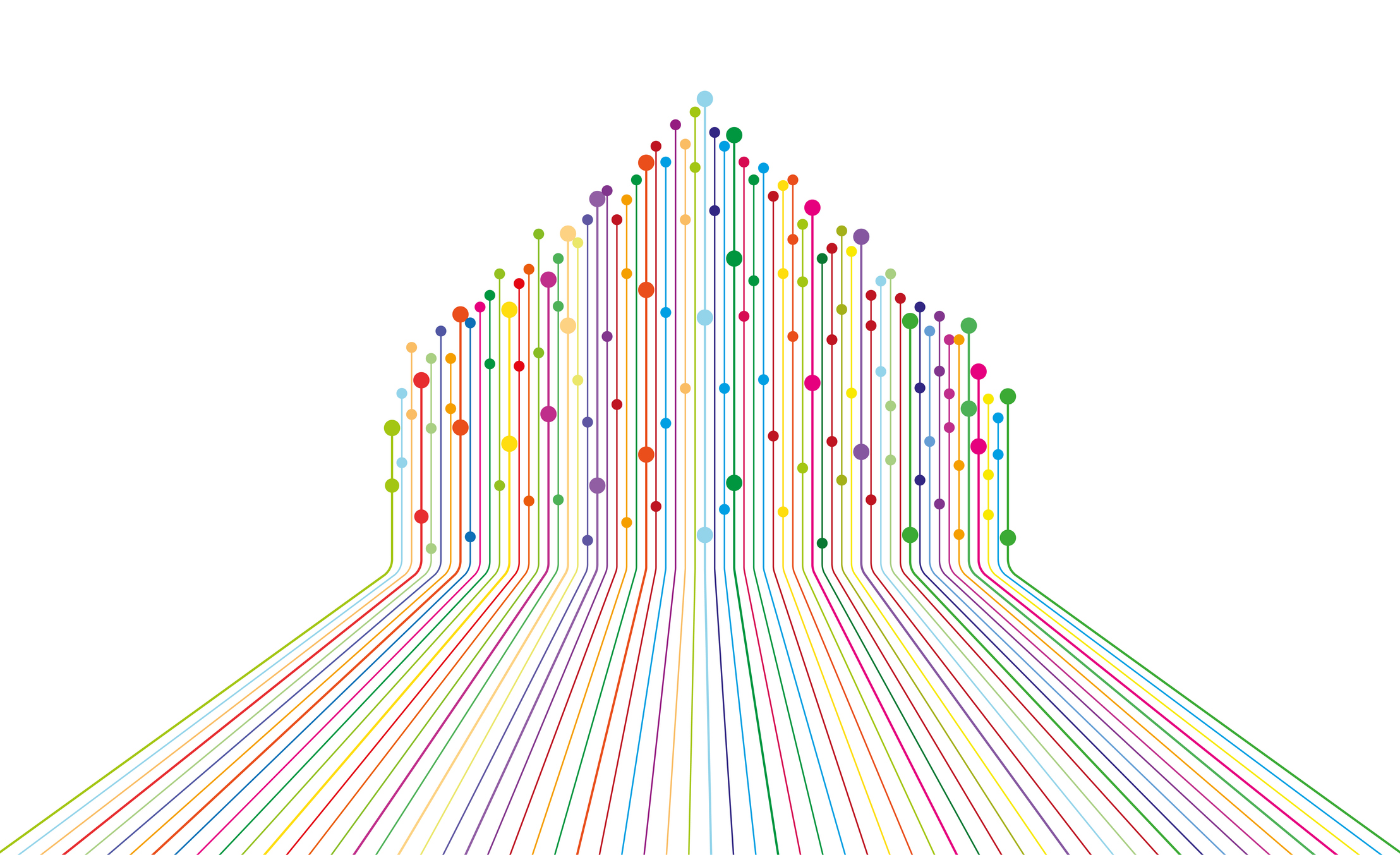
Unlocking the potential
of Data & AI
via Pivotl’s Data
Platform Strategy
In the era of AI, data is critical to business innovation and efficiency. Organisations that can make use of the potential of their data gain a competitive advantage. They can streamline operations, make informed decisions, and provide more intelligent, targeted services to their customers.
The key question for enterprise scale organisations to ask themselves is how they go from amassing more and more unused data to leveraging it more effectively.
I believe the key to success with data and AI is in having an effective data platform strategy.
In this blog post I introduce the concept of data platforms, why they are needed, and what to consider. A first in a series of blogs unpacking data platforms and tools in more detail.
how enterprise organisations go from amassing more and more unused data to leveraging it more effectively.
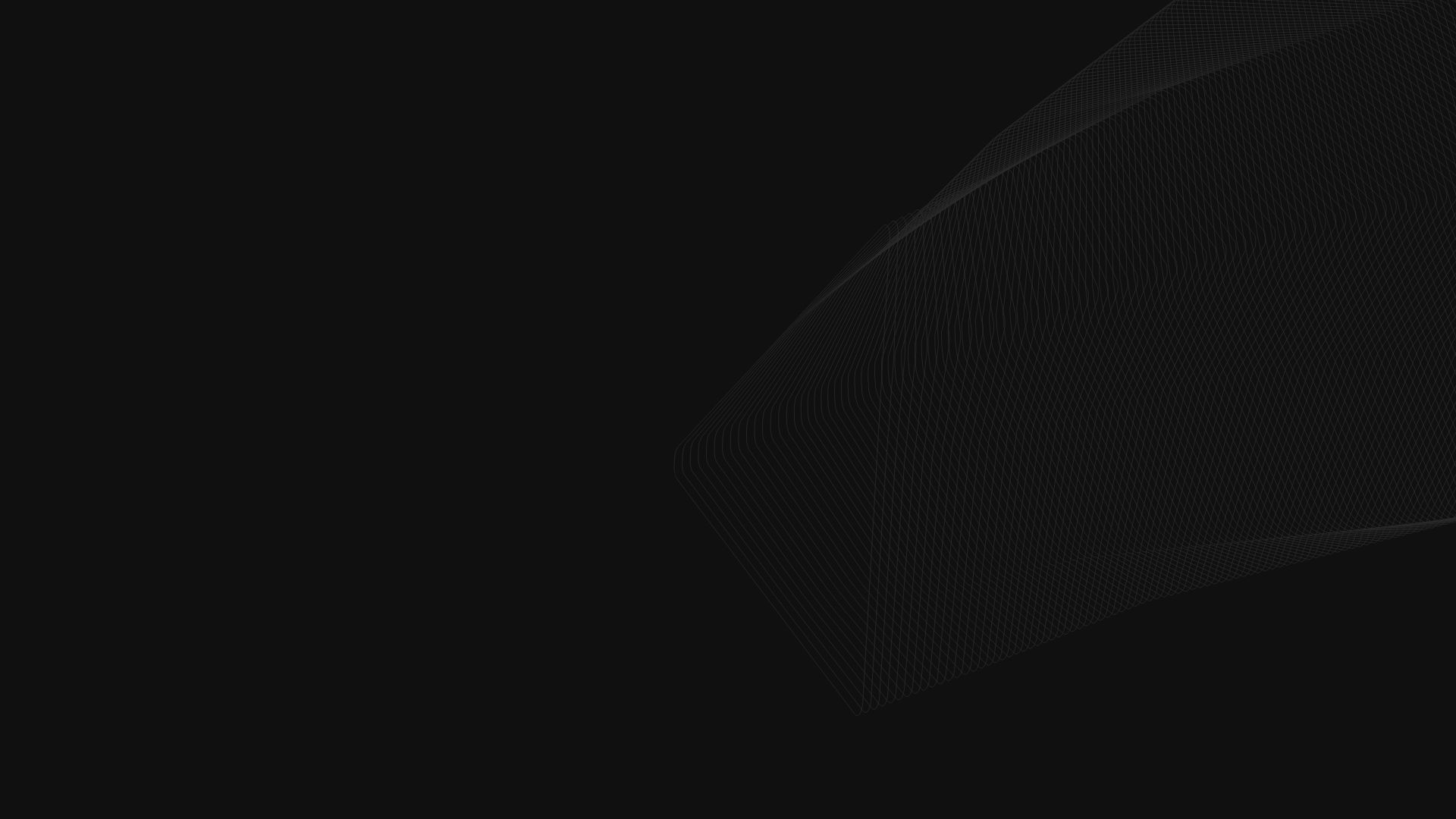
introducing data platforms
I’ve worked with many large organisations in both the public and commercial sectors, and there is a common theme; most struggle with the volume and diversity of data and cite it as one of the most important strategic challenges.
5 key challenges
Data availability
Data is not readily available, or not in the right format to be useful, which creates organisational blind spots. A typical manager’s complaint is ‘why is it so hard to find out’.
Data quality
Staff may be unable to trust available data to make decisions, despite the last few strategies emphasising becoming ‘data driven’. This can lead to ‘off-piste’ hacks, using data sources they do trust to make their management decisions.
Data and tools silos
Data solutions tend to exist in pockets. Typically, these pockets fall into types: some are held on premise (i.e., in an organisation’s own software and hardware set-up), some are ‘legacy’ (i.e., an old solution that the organisation still relies on – regardless of its suitability, modernity and performance), and some up-to-date ‘cloud solutions’ where the data sits on well-supported third party platforms, delivered as a remote service. This typical set-up relies on an array of different technology solutions, which is a real problem to support and maintain. Different staff and expertise can be required to attend to each variant, adding a cost and management consideration.
Scaling and availability
There is often an inability to scale up data solutions to support higher volumes and velocities of data i.e., supporting real-time use cases, new product launches, new office openings, and so on. Equally, legacy set-ups can mean that scaling down or deprecating as the need arises can be a long-term (and costly) decision. This makes forecasting and budgeting difficult.
Integration
Easily breakable point-to-point or custom integration solutions that frequently fail due to the demand to support different types and formats of data can collapse any system. Unless data sources can integrate, this is another potentially ruinous overhead.
Modern, cloud-based data platforms were introduced to solve some of these problems, among others.
Pivotl believes that hyper-scale cloud services can provide the answer to most typical use cases in efficient data management and exploitation. There are several key reasons for this:
- Cloud software and hardware offer, from a single console, sophisticated storage, database, compute and AI capabilities
- Costs track against usage – rather than a fixed estimation
- Consolidation around the major cloud vendors has meant that skills are readily available, and kept up to date
- Security, scalability, costs and redundancy (where you can failover to new machines) all compare favourably to on premises and legacy solutions
- Even in industries requiring the most stringent private environments can now rely on cloud companies to provide locally domiciled and secure computing
- Importantly, AI functionality can be sourced from the cloud companies with no need to procure separately, with solutions covering mapping, machine learning, image recognition, intelligent design and more available from a single console.
The term ‘platform’ can be confusing – and is often misused. When we say data platform, it could mean one of the following:
- On premise data platform
- Hybrid data platform
- Bespoke data platform (made up of different modern data stack tools)
- Cloud native data platform
Our focus is on cloud native data platforms, such as Azure and Databricks, but these are of course not always the right answer. As a Microsoft partner, we help clients learn what is available with minimal start-up overheads.
Prior to the modern data stack, data solutions like Hadoop, for example, followed an extract, transform, and load (ETL) design that meant data was transformed or changed before it was stored, which was often slow and difficult to scale.
The evolution of this design was to invert ETL to become ELT or in other words loading data into a data lake before it is transformed, which has the advantage of easily supporting different data formats/types and being highly scalable. See example Microsoft reference architecture for the data lake design.
The term ‘modern data stack’ typically describes the cloud-enabled ELT design pattern and encompasses a variety of different tools from different vendors that make up parts of the data platform e.g., ingestion tools or transformation tools like Fivetran or Atlan.
A data platform is a set of components, which data flows through (data lifecycle).
It is a comprehensive ecosystem that consolidates data from various sources, processes it, and makes it accessible for analysis and decision-making. It integrates multiple technologies and tools to ensure that data is ingested, stored, managed, and analysed efficiently and securely.
Data platforms, such as Azure Synapse/Fabric or Databricks and their constituent components, need to be configured to meet the specific needs of an organisation.
The term ‘modern data stack’ typically describes the cloud-enabled ELT (Extract, Load, Transform) design pattern
We see these as the constituent components of a data platform:
- Data ingestion: The process of gathering raw data from various sources, such as databases, applications, open data, and external data feeds.
- Data storage: Securely storing data in a scalable manner, often using data lakes, warehouses, or hybrid solutions e.g., lakehouses, SQL databases (for tabular data), NoSQL databases (for non-tabular data, images, files, etc)
- Data processing: Transforming raw data into usable formats through transformation processes, real-time streaming, and batch processing.
- Data management: Ensuring data quality, governance, and compliance through comprehensive management practices.
- Data analysis and AI: Providing tools and interfaces for querying, analysing, and visualising data to derive actionable insights or using data to support AI use cases
- Data presentation: Showing the data to users in an appealing, interactive format – which for Microsoft users might be via Power BI, which links to the layers above.
Organisational benefits of a cloud Data Platform
- Centralised data management: A unified platform eliminates data silos, providing a single source of truth and improving data accuracy and consistency.
- Decision making: By making data readily accessible and easy to interrogate, organisations can make informed, accurate decisions more quickly.
- Scalability: Modern data platforms, such as data lakes and lakehouses, are designed to scale as data size grows and velocity increases, ensuring performance and reliability at scale.
- Cost efficiency: Efficient data processing and storage solutions reduce operational costs and avoid resource wastage, which when aligned closely with your cloud strategy can unlock significant value from your cloud subscription.
- Enabling AI: Access to a breadth of high-quality data assets helps organisations to exploit new business opportunities created by advancements in AI.
A well-defined data platform strategy, aligned to your organisational context, is essential for unlocking the full potential of your data and enabling effective AI initiatives
Why you need to define your Data Platform Strategy
Designing an effective data platform strategy is a multifaceted effort that requires expertise across various domain areas, such as cloud, cyber, data, and AI. It is also a process, rather than a single step.
Consider the following against your organisation’s priorities as you consider moving to a cloud platform set-up. Each comes out of the box with Microsoft Azure:
- Managing complexity: The complexity of integrating different, disparate data sources, technologies, and processes requires special knowledge and experience. Note; It is important that your data platform strategy reflects the simplicity or complexity of your data and AI use cases.
- Customisation: Every organisation has unique data needs and goals with differing resource/cost constraints and preferences. A one-size-fits-all approach won’t work, so tailoring your platform strategy to your specific requirements is crucial.
- Best practices: Staying ahead of the latest industry trends and best practices ensures that your data platform is future-proof and leverages robust yet cutting-edge technologies.
- Cost optimisation: Expert guidance helps in selecting cost-effective solutions and avoiding unnecessary expenditures. See our post on cloud cost optimisation.
- Security and compliance: Ensuring data is managed in compliance with regulations and protected against breaches is crucial. Security experts can navigate the complex landscape of data security and compliance.
closing thoughts
A well-defined data platform strategy, aligned to your organisational context, is essential for unlocking the full potential of your data and enabling effective AI initiatives. It has the potential to streamline and consolidate your architecture, particularly when aligned to your cloud strategy, and alongside a data strategy and methodology, it can act as a catalyst to help resolve the data challenges being faced by a lot of organisations.
In a future edition of my blog series, I explore how you can devise a data platform strategy for simple analytics use cases through to more complex big data and AI/ML requirements. I will also unbox leading data platforms from Azure (showing different configurations) and Databricks to explore their optimal use cases and pros/cons.