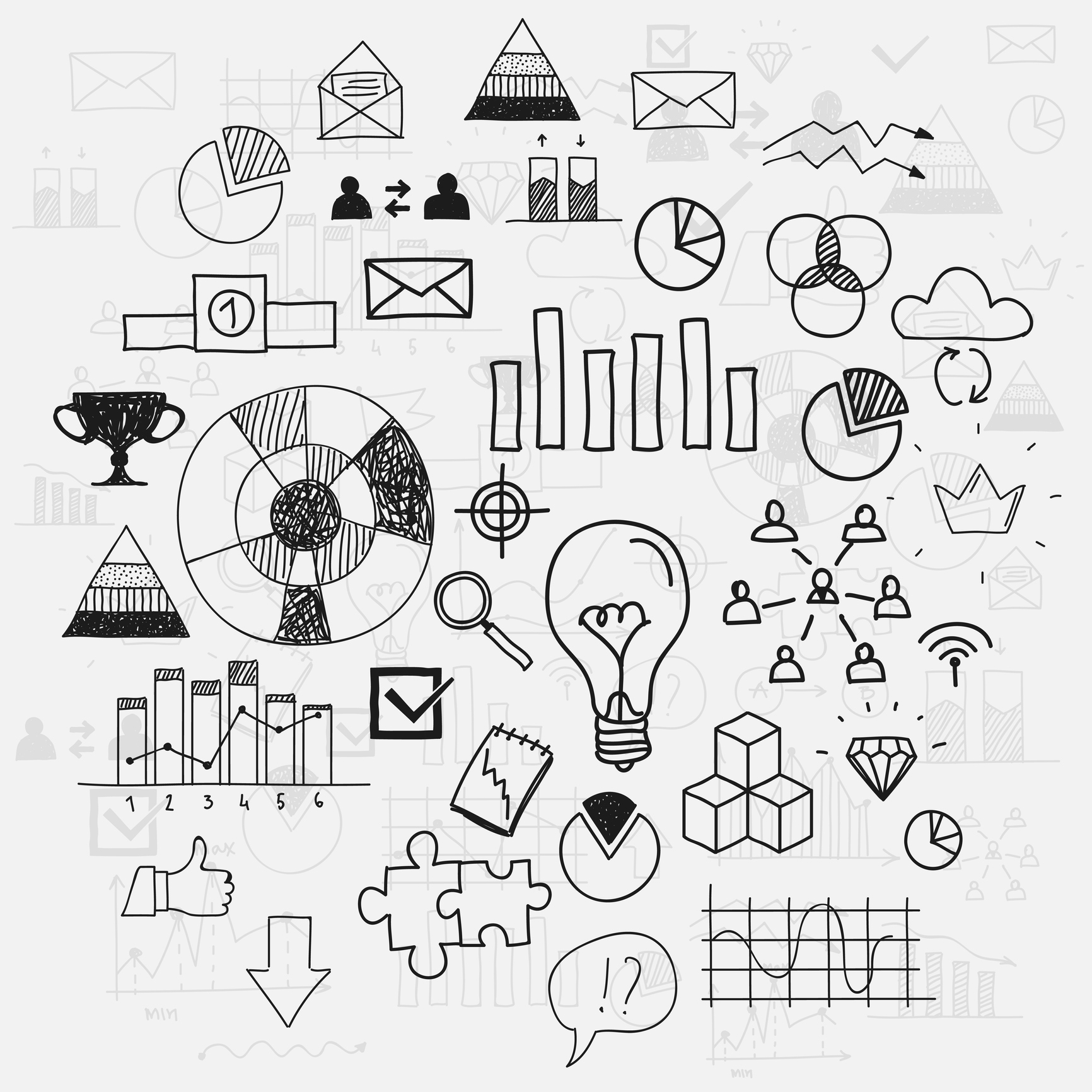
Data Learnings from
Pivotl’s first few months
I was stuck for six hours at Gatwick on Saturday on my way to see the marvellous Julia Glidden speak at the Tallinn Digital Summit and Open Government Forum in Estonia. So, I thought I’d use the time productively to jot down some hazy, late summer reflections on the state of the data transformation market.
We’ve been at it for about 6 months at Pivotl, thinking, writing and talking to all sorts of people grappling with the challenge and opportunity of doing more with data.
I’d be fascinated to hear if your experiences are the same. Email me or join the conversation on LinkedIn.
Actions Speak Louder than Words, but Not Nearly as Often
Since starting Pivotl we’ve noticed common themes as to why organisations engage us – policy changes requiring data ingestion, migrations, database modernisation, fixing broken data pipelines, data training, preparing cloud for data, AI / ML experimentation, dash boarding, visualisation and data science Proofs of Concepts.
Notably absent though are requirements generated by business driven, detailed data centric strategies that encompass and require change across the entire organisation.
Notably absent are requirements generated by business driven, detailed data centric strategies that encompass and require change across the entire organisation.
Searching for Business Outcomes
Data is such a broad term as to be almost unhelpful – it’s possibly too abstract even as a high-level call to action.
Across all sectors C-Suite Executives know to talk about the importance of data and to reference it in annual plans, policy documents and speeches. But there is limited maturity in the boardroom in terms of cogently linking data to business goals and identifying all aspects of business change required to truly exploit data.
The connective tissue is not there yet.
Lack of Pathway
To be fair to the Executive class there is a lack of clear governance and funding pathways to enterprise-wide data centricity.
For example, in government, if you want to (re) build online public services via a digital transformation program there’s a very clear spend, procurement, supplier, project methodology route – no one got sacked for following the service manual and hiring Deloitte.
But with data, the next steps, even if you have a decent strategy, are less clear. Effective change requires clear next steps and well described destinations.
Scaled exploitation of data requires wider workforce participation.
Data isn’t Digital
Meaningful exploitation of data, at scale, so that humans and computers can make better, faster, more evidenced based decisions isn’t the same as the current interpretation of Digital Transformation.
Sure, there’s a lot of adjacencies – cloud, software, product thinking – but it’s not the same. It requires different leadership, refreshed approach to technology, project methods, talent, suppliers and, crucially, it’s a whole team sport. Online user experience can be largely transformed whilst leaving the analogue core of an organisation undisturbed.
Scaled exploitation of data requires wider workforce participation.
Agile is a Challenge for Data
Move fast and break things was a useful mantra for consumer facing product teams looking to attract an audience rapidly and iterate based on their needs.
In this rush to exploit the elasticity of the cloud and modern engineering practice data governance, provenance and quality has degraded. The modern data stack is a partial answer to this but as always with tech solutions – there are wider human changes that need to happen to make this work.
there are wider human changes that need to happen to make this work.
all transformation to come will be built off the back of well curated, managed and shared data.
Data is the Only Certainty
Most boards are taking a sensible approach to Generative AI and Chat GPT; not completely ignoring it as so many did with public cloud, but also taking time to work through the hype to understand what it means for their organisational context.
We don’t know yet what LLMs mean for a council or a utilities company. But one thing we do know is that all transformation to come will be built off the back of well curated, managed and shared data. This is something that we can get on with whilst running (G)AI experiments.
Data Ages Like Fine Wine, Applications Like Fish
This is the most exciting thing for me. The art of the possible with modern data platforms, tooling and practice feels like it removes some of the pressure from applications.
In other words, migrating off legacy applications still matters but the smart abstraction of data from the application layer means that perhaps we don’t have to always wait to migrate off applications to enable transformation.
That’s a big mindset shift, though, and requires a lot of change to happen.
What’s been your experience?
I would love to know if this reflects your experience (join the conversation on LinkedIn) and, of course, if you want help with any of it – drop me a line, we love talking about this stuff.